Communication and Technology Trust
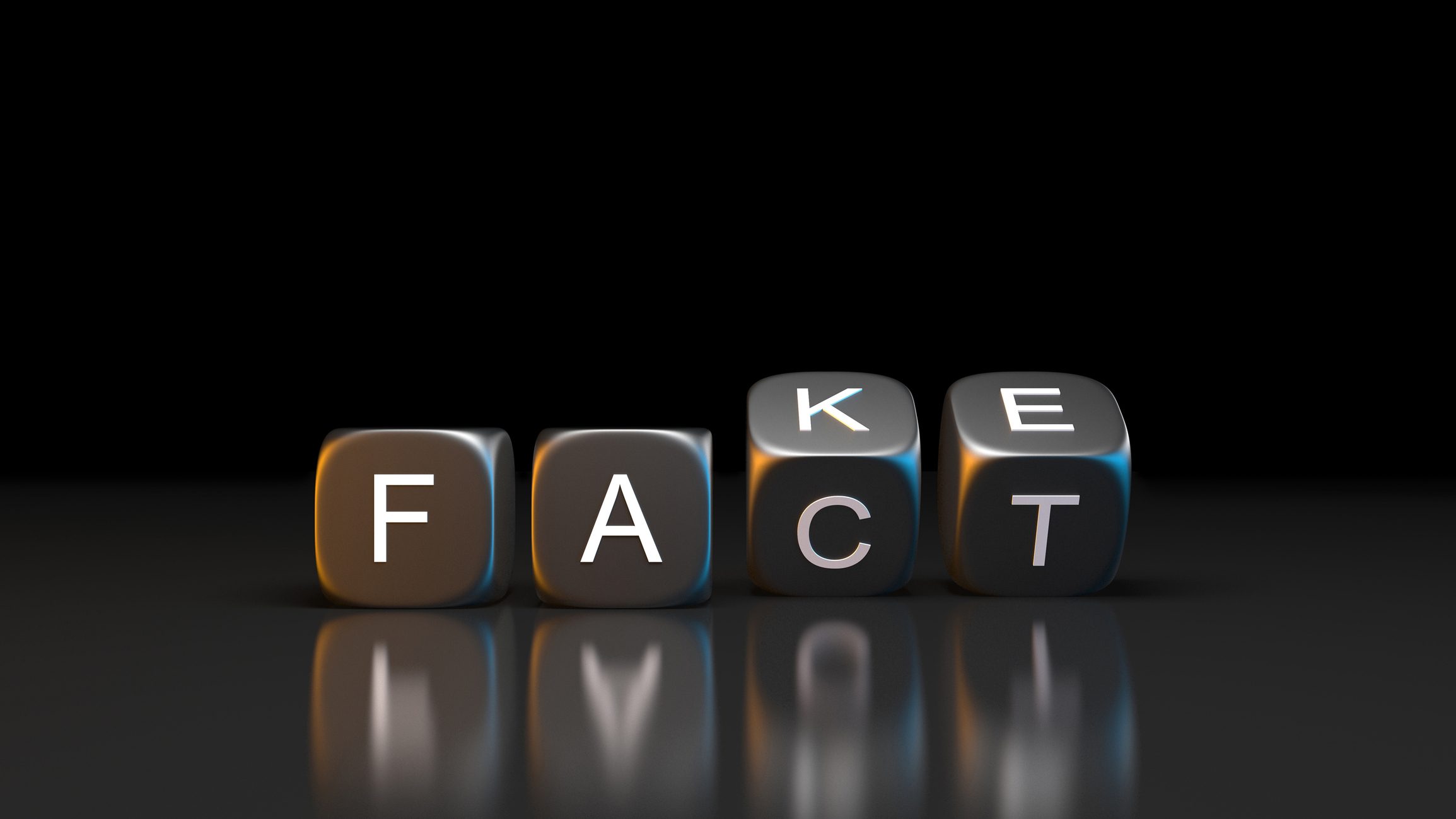
Dr. Jieun Shin: Algorithms and Their Impact on Misinformation
CJC Telecommunication Assistant Professor Jieun Shin was interviewed on Nov. 10, 2020 about her research on algorithms, AI and the spread of misinformation.
Below the video is an edited transcript of that interview.
Much of your recent research has focused on algorithms and the spread of misinformation. How did you become interested in this topic?
Shin: I believe in general there are two different ways that people consume information. One is choice, and the other is chance. My research in the past has looked at how people are motivated to look at certain information and one of the most popular mechanism is selective exposure. People are politically motivated to read a certain message over the other. People have a motivation and they make a choice to read a message A over message B. But I think what is becoming more and more interesting and more important is how people are forced to make their choices. That is a chance. What is available around them and recommendation algorithms and search algorithms and also who they are connected to on social media makes that information possible.
I wanted to focus more on this choice problem. How people encounter information in their natural digital media environment. That’s how I became interested in the algorithms.
For the Consortium on Trust in Media and Technology, you are exploring the creation, distribution, and combating of misinformation and how human and machines relate to each other. Tell us about that research and what you’ve found so far.
Shin: That’s a good description of my research because digital media is an intricate ecosystem where humans and machines interact and their response to each other’s action creates the entire dynamics. For instance, in the diffusion and the misinformation creation stage, we have noticed that bots play a critical role. They find this misinformation and they inject this big news and misinformation into the social media platform. And they tweet and they make it available, they share these messages. You might wonder, even if there are millions of bots on social media and they tweet multiple times, what’s their effect if nobody pays attention to them? They could be harmless but the problem is that, unfortunately, people respond to those messages.
There is a phenomenon called the illusion of truth. Even though people believe that something may not be true, if they hear it repeatedly, because it sounds familiar, they tend to believe it because familiarity breeds credibility. And even though it might be a small group of people, if they retweet it, think about all the people connected to them. They are their friends, they are the people who they know and trust and that’s how this misinformation spreads and trickles down to the regular people.
Of course, we cannot forget that some people share this unverified sources because they want to show that they are in the know, they know more novel information than the others and some people just show that they are partisan. They are cheering for their party, their candidate.
The bots play a critical role in the diffusion stage. But when it comes to fighting misinformation, actually the interaction between humans and bots and machines become more evident. This is why. Usually, fact checking happens through human endeavor. There is intensive human labor involved in this process. But only a small group of fact-checkers exist, so to tackle this sheer amount of misinformation that is being produced every day is impossible.
Computer scientists and programmers are trying to develop an AI system that automatically detects misinformation based on certain features. We don’t know exactly what’s going on unlike regression models for social scientists, where we carefully put in certain parameters and variables into the model. But a lot of times this machine learning and AI, this algorithm detects features that are salient to them but it’s sometimes very muddy and it’s invisible to human eyes. Even those who created those algorithms are not sure about what outcomes are produced from all these programs. They really have to depend on the data. They literally are fed by the data and they find some patterns that looks like a misinformation.
This algorithm that detects misinformation plays the role of a policeman. But the bad actors are also using AI. The bots are also AI. They learn from the policeman’s behavior. The more they get caught, certain behaviors are detected, they learn quickly, they adapt. AI, by definition, is any program that learns and adapts. Therefore, bots learn and adapt to the new environment. They constantly change their behavior so that they don’t get caught. That’s the problem. They both constantly evolve, they try to outbid each other, but the challenge lies right there. It’s difficult to eradicate misinformation or bad actors completely.
What are you hoping that people will do with that research in order to address this problem?
Shin: So far I have looked at how misinformation spreads on social media and digital platform. But one concern that I have with my research is that once you emphasize that there all these bad actors, it lowers our trust in media systems altogether. If you argue that you shouldn’t trust anybody, that means they’re going to be skeptical of experts and those good quality sources too. We need to make a distinction between bad actors and good actors. Of course, information consumers are overwhelmed. They cannot make a cognitive effort to determine what is true and what is not? Therefore, we need to make it more efficient for people to identify which one is a good actor. At least which one passes the bar.
As far as I know, there are a few projects that are still under development and the ones that are publishing their measurements include NewsQ and NewsGuard. They measure the quality of new sources based on their criteria, whether they repeatedly published false information or they separate false information and also whether they clearly disclose financial motivation, the ownership, and whether they separate opinions and news and all those things, so they have their own criteria and they compute the binary score of what they call quality. Of course, we need to discuss intensively how we are going to measure the quality of information. One thing that we need to constantly strive for is better measurement, but it’s out there. We need to make it more available, publicly displayed so that people can make efficient decisions.
Will this research provide direction for social media companies on how they can play a role in minimizing misinformation?
Shin: The reason why I looked at the Amazon algorithm is that this architecture and these roadmaps are important for people to encounter good information. I believe the more consumers demand higher standards, the more social media companies will respond.
In the beginning, Facebook, Twitter, and others argued that they are just platform companies. They are not media companies. They have nothing to do with the misinformation. But now they have changed their position. They’re trying to moderate. It’s because they’re under pressure from media social media users have demanded it. Therefore, we need to find out how their algorithm work and put pressure on them to make it more transparent.
Another area of your research is the development of public interest-minded recommendation algorithms for large platform companies like Amazon. What is the intent of that research?
Shin: The priority for platform companies is to increase a customer’s engagement. The more they click on their pages, the more items they purchase, the more eyeballs they get. Unfortunately, public health and public interest is not their priority. Even though they don’t promote misinformation, the algorithm itself is designed to promote eyeballs and engagement. They want people to spend more time on certain pages and certain products. Therefore, their algorithm takes into account the features that are not relevant to public interest. We’re asking them to design their algorithms to be publicly minded.
Posted: January 10, 2021
Tagged as: AIatUF, Algorithms, Jieun Shin, Misinformation